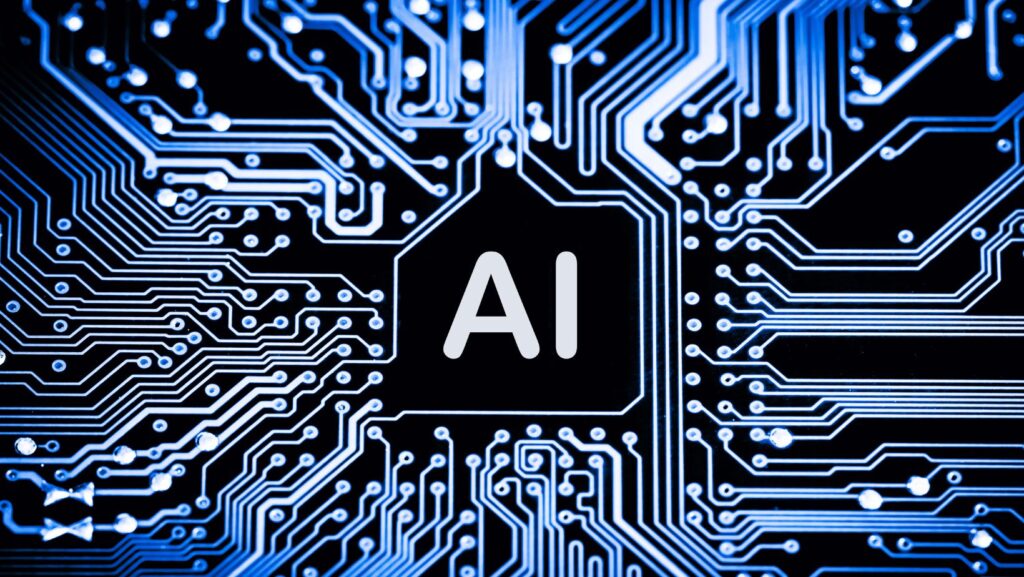
The environmental impact of AI is an increasingly relevant issue as technology advances at a rapid pace. Machine learning models require substantial computational power, which in turn demands significant electricity consumption. While some entities are completely discouraging the use of technology, others choose a more moderate approach to AI and other tools.
Beyond electricity consumption, the infrastructure supporting AI involves extensive resources that can strain the environment. The data centers housing AI servers not only consume vast amounts of energy but also considerable water resources, leading to sustainability challenges. These facilities generate electronic waste, contributing to pollution and resource depletion issues.
AI’s environmental footprint isn’t limited to direct energy usage. The metals and materials used in AI hardware production can lead to soil erosion and pollution when not carefully managed. These factors underscore the need for improved environmental practices and policies to mitigate AI’s impact. Understanding these elements is crucial for you to appreciate the broader implications of AI development on our planet.
Direct Environmental Consequences of Artificial Intelligence
Artificial intelligence has a notable environmental impact primarily due to its significant energy consumption and carbon emissions. Data centers housing AI systems also demand substantial resources, contributing to electronic waste generation.
Energy Consumption of AI Systems
AI systems require vast amounts of energy, especially during the training and operation phases of deep learning models. High-performance computation and extensive data processing amplify the electricity demand. As algorithms become more complex, the need for more powerful hardware and energy-intensive processes increases.
A prominent example is the use of GPUs and TPUs, which are designed for rapid processing but consume large power outputs. This heightened energy usage often relies on non-renewable energy sources, which contributes to their environmental footprint.
Efforts are being made to improve efficiency through optimized algorithms and hardware innovations. Nevertheless, the challenge remains enormous as AI technologies expand across various industries and applications.
Carbon Footprint of Machine Learning Models
Machine learning models, especially those involving deep neural networks, contribute significantly to carbon emissions. Developing and deploying these models involve substantial computational resources, which often run on fossil fuel-powered electricity grids.
One analysis showed that training large transformer models can emit as much CO2 as five cars over their lifetimes. This underscores the urgent need for strategies that mitigate the carbon footprint.
Optimizing model architectures and employing energy-efficient data centers are methods being explored. By addressing these concerns, AI can potentially become a more sustainable technology.
Data Center Resource Usage
Data centers, which host AI system servers, demand substantial resources. These facilities account for a significant portion of global energy usage and contribute to electronic waste due to frequent technology updates.
Water usage is another concern. Data centers often consume large volumes of water for cooling purposes, particularly in regions where water scarcity is increasingly problematic. Over time, the expansion of data center infrastructure can exacerbate resource depletion.
To address these issues, companies are exploring renewable energy sources and improved cooling methods. The goal is to minimize environmental impact while maintaining the scale of operations required by growing AI applications.
Broader Environmental Effects of AI Implementation
The integration of AI in various fields brings substantial environmental challenges and opportunities. Its influence extends to resource management, electronic waste, and shaping policies.
AI in Resource Management and Conservation
AI technology plays a vital role in enhancing resource management and conservation efforts. It helps in predicting natural resource availability and identifying optimal conservation strategies. Sensors and AI algorithms track wildlife, monitor deforestation, and assess water quality. This leads to more efficient intervention strategies.
Additionally, AI-driven models aid in forecasting weather patterns and climate changes. This helps agricultural planning and disaster management, ensuring better preparedness. The use of predictive analytics contributes to creating efficient systems for resource allocation and consumption reduction.
Electronic Waste and Hardware Lifecycles
The proliferation of AI systems significantly contributes to electronic waste. Data centers, which are essential for AI operations, generate a high volume of obsolete hardware. Components such as servers and storage devices are regularly upgraded, resulting in significant e-waste.
Proper management of the hardware lifecycle is essential. Recycling and reuse programs can mitigate the adverse effects of e-waste, preserving valuable materials and reducing environmental harm. Stakeholders must consider sustainable disposal practices to curb the negative impacts.
Impact on Environmental Policy and Regulation
AI’s role in shaping environmental policy is growing. It provides policymakers with robust data and models that inform decisions on environmental regulations. By analyzing vast datasets, AI can assist in predicting environmental outcomes and setting realistic goals.
Policies that address AI’s environmental impact, such as reducing its carbon footprint, are becoming common. These regulations encourage the development of sustainable AI practices, promoting eco-friendly advancements in technology. The integration of AI in policy-making can drive substantial improvements in environmental conservation efforts.